Introducing HPT: A Groundbreaking Family of Leading Multimodal LLMsMarch 19th, 2024 - HyperGAI Team
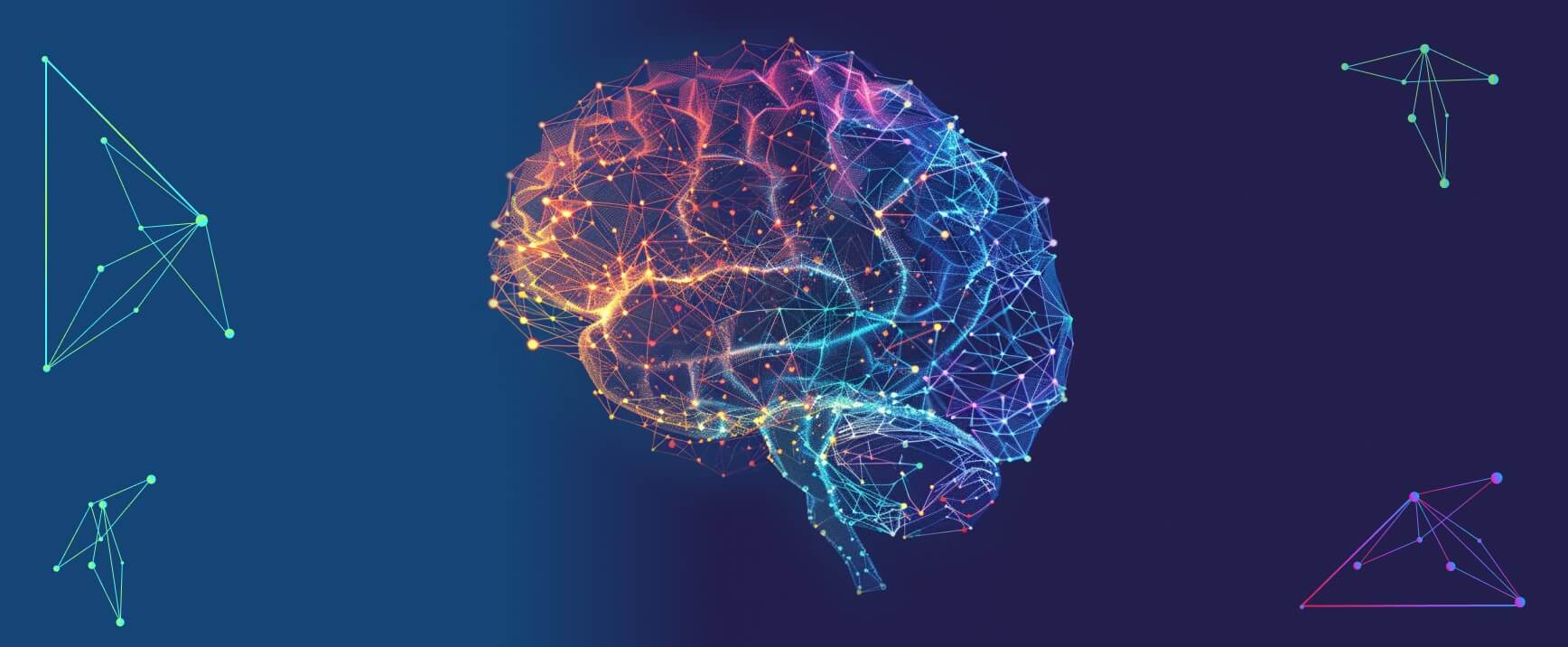
What is HPT and Why is it exciting?
Multimodal large language model (Multimodal LLM) is a type of Multimodal Foundation Models that are the cornerstone towards building Artificial General Intelligence (AGI). Unlike the conventional text-only LLMs, Multimodal LLMs aim to understand more than just text, including multiple modalities of input such as text, image, video, and more.
We propose a novel multimodal LLM pretraining framework named “Hyper-Pretrained Transformers” (HPT), which is able to train a large multimodal foundation model that is capable of understanding multiple modalities of inputs in an efficient and scalable manner.
The proposed HPT framework can be either trained from scratch or adapted efficiently with existing pretrained vision encoder and/or pretrained large language models.
We‘ve built our first version of HPT, HPT 1.0, which come with two different model sizes:
- HPT Pro — our most capable model for solving very complex multimodal tasks, and
- HPT Air — our most efficient model as a cost-effective solution that is capable of solving a wide range of vision-and-language tasks.
The key highlights and features of HPT include:
- HPT is a general new framework for training next-generation multimodal LLMs and can be easily extended with any other existing pretrained models in a scalable way,
- HPT Pro outperforms other larger proprietary models like GPT-4V and Gemini Pro on the MMBench and SEED-Image benchmarks, and achieves the state-of-the-art results for among the models of its similar sizes in the MMMU leaderboard, and,
- HPT Air is publicly available and achieves state-of-the-art results among all the open-source multimodal LLM models of similar or smaller sizes on the challenging MMMU benchmark.
How does HPT work?
HPT aims at training a multimodal foundation model adept at a broad spectrum of complex vision-language understanding tasks, including performing deliberate reasoning, analyzing charts, diagrams, natural imagery, and more. The H-Former, the innovative feature of HPT, serves as a bridge between the vision and language modalities by converting visual data into language tokens. This enables the LLMs to comprehend visual contents despite being pretrained on text exclusively. To this end, H-Former integrates a dual-network design to learn both local and global features for vision-language alignment, allowing HPT to understand both fine-grained details and abstract, high-level information. Figure 1 illustrates HPT‘s overall model architecture.
In principle, HPT can either train from scratch or leverage existing pretrained vision and language models. For our open-source HPT Air model, we leverage a pre trained LLM and vision encoder, further train it on a multimodal training dataset with only about 1.6M text-image samples where the text content is exclusively in English.
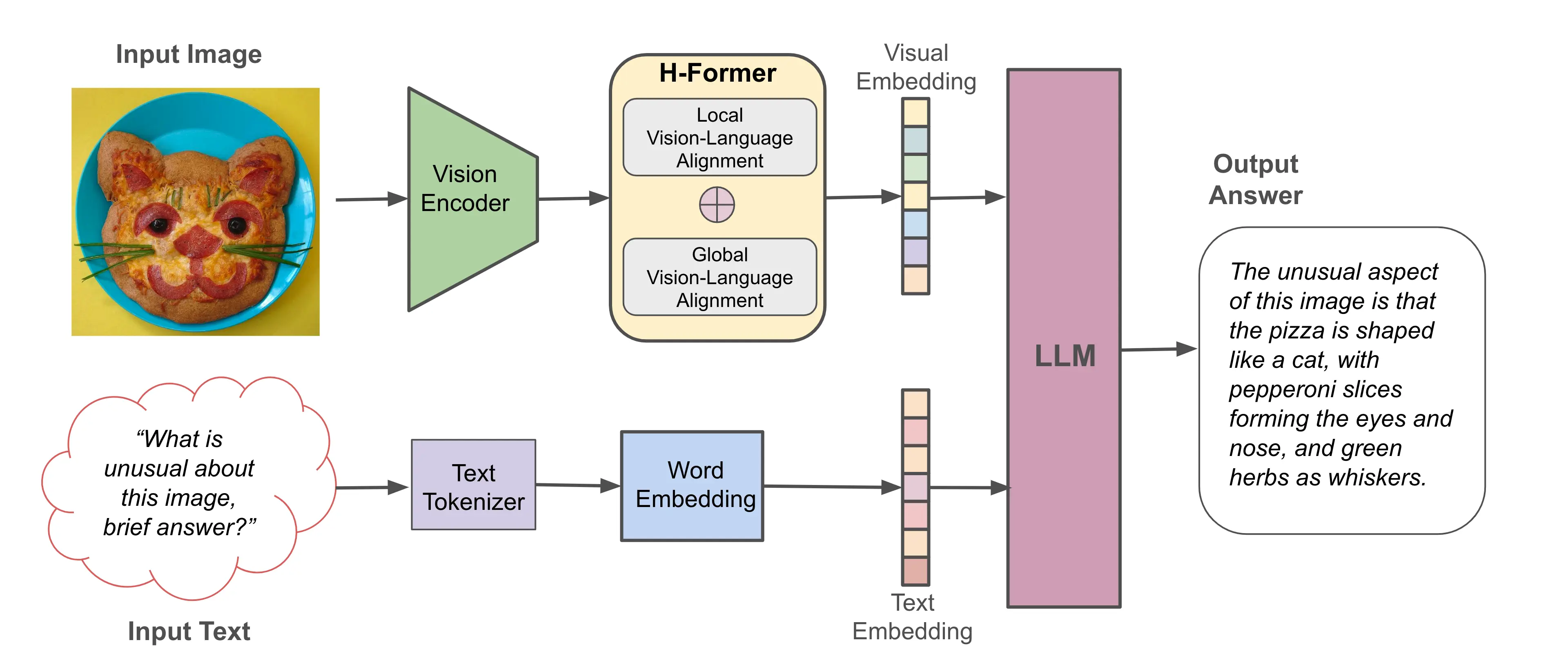
Figure 1. An illustration of the HPT model architecture
Strong Performance on Multimodal Benchmarks
We evaluate the empirical performance of our HPT models for their multimodal understanding capabilities on a suite of multiple challenging multimodal benchmarks. The selected benchmarks require either college-level subject knowledge and deliberate reasoning in multidisciplinary areas (MMMU and CMMMU), or common-sense and spatial understanding in various vision and language tasks (SEED (img), MMBench, and MMBench-CN). Collectively, they encompass a wide range of image categories, including charts, diagrams, portraits, and photographs. This comprehensive evaluation ensures that our models are adept at interpreting and reasoning across a multitude of visual and textual scenarios.
The benchmarking questions can be either in multiple choice (MCQ) or open-ended question formats. We follow the official evaluation procedure and match the model‘s answer exactly with the ground truth for MCQ and follow their soft matching strategies for open-ended questions. Table 1 reports the official match rates on the benchmarks considered, higher is better.
In many cases, HPT Pro and HPT Air demonstrate superior performances against leading contenders like GPT-4V, Gemini Pro, and Qwen-VL. For example, on SEED (img), HPT Pro achieved the best result (73.1%) across all competitors while HPT Air outperformed Qwen-VL-Chat (69.7% vs 65.4%) and even reached Gemini Pro performance (69.7% vs 70.7%). A similar conclusion can also be observed on MMBench and MMBench-CN, where the one exception is LLaVA-NeXT which outperformed HPT Air on SEED (img).
On the challenging MMMU and CMMMU benchmarks that require college-level subject knowledge and deliberate reasoning, HPT Pro and HPT Air are the best among the models of their sizes, respectively (the snapshot of the MMMU leaderboard is available at:https://mmmu-benchmark.github.io/#leaderboard). Lastly, despite being trained exclusively in English based multimodal data, our HPT models can generalize well to other languages such as Chinese as demonstrated by the competitive results on the MMBench-CN and CMMMU benchmarks.
Benchmark | Description | Proprietary | Open-source (<10B) | ||||
---|---|---|---|---|---|---|---|
HPT Pro | GPT-4V | Gemini Pro | HPT Air | LLaVA-NeXT | Qwen-VL-Chat | ||
MMBench | Multimodal understanding in English | 77.5% | 77.0% | 73.6% | 69.8% | 68.7% | 61.8% |
MMMU(val) | College-level understanding and reasoning in English | 52.0% | 56.8% | 47.9% | 44.0% | 35.3% | 35.9% |
SEED(Img) | Visual perception and understanding in English | 73.1% | 69.1% | 70.7% | 69.7% | 72.2% | 65.4% |
MMBench-CN | Multimodal understanding in Chinese | 76.7% | 74.4% | 74.3% | 70.1% | 61.2% | 56.3% |
CMMMU | Colleage-level understanding and reasoning in Chinese | 39.2% | 43.7% | / | 31.7% | / | 31.3% |
Table 1: Evaluation of our HPT models compared with other competitive models on multiple multimodal benchmarks. Note that the bold and underline numbers indicate the best and second best results among the compared models on each benchmark category, respectively.
Qualitative Examples of Multimodal Capabilities
In the following, we show some real qualitative examples to demonstrate several interesting multimodal capabilities emerged from HPT.
- See, describe, and follow instructions. HPT can comprehend and articulate the contents of visual images. Despite the unconventional nature of the images in examples 1.1 and 1.2, HPT accurately discerns the primary subject and articulates its characteristics. It is worth noting that example 1.1 is similar to the one featured in our Figure 1. HPT can tailor the verbosity of its descriptions to user preferences, delivering concise responses when prompted with the keyword “brief answer.“ This feature ensures that HPT can follow instructions to meet the user‘s demands.
- Interpret and reason. HPT is adept at interpreting charts, diagrams, and responding to scientific questions grounded by the data presented. For instance, in example 2.1, HPT demonstrates its capability to recognize a numerical sequence - 76, _, 68, 67, 58—as corresponding to chart data, enabling it to deduce the missing number through chart analysis. In example 2.2, HPT can answer the college-level question and provide the correct explanation. These examples illustrate HPT‘s proficiency in extracting and applying information from visual data to solve complex problems.
- Understand conceptual art. HPT also excels at grasping artistic expressions, accurately interpreting the emotions conveyed in example 3.1, and recognizing the cultural significance of the Merlion as a symbol of Singapore in example 3.2. This capability demonstrates HPT‘s proficiency in analyzing and understanding complex visual and conceptual cues within artwork.
- Being creative. HPT is not only strong in understanding, but also highly creative. As shown in example 4.1, it not only understands that smartphones didn‘t exist during Einstein time, but also can creatively speculate on the possibilities had he access to modern technologies. Furthermore, HPT accurately interprets pronoun references, as seen in the second question where it correctly identifies “he“ as Albert Einstein and responds appropriately. This highlights HPT‘s nuanced understanding and imaginative reasoning. HPT can also write an amusing fiction story based on the provided image as shown in example 4.2.
- Recommend and assist. HPT can also provide useful recommendations. Despite the tricky visual appearance in example 5.1, it understands the image content, recommends a dish that can be prepared using such ingredients, along with places to enjoy such meals.
Similarly, based on your image, HPT can help plan your next cruise ship and provide recommendations to alleviate seasickness (example 5.2). This showcases HPT‘s ability to provide actionable insights and useful recommendations to enhance user experiences.
The Bottom Line
We have developed HPT as an innovative framework for multimodal LLM pre-training. HPT offers a versatile and scalable framework to build multimodal foundation models that are proficient in understanding multiple input types such as text and and vision. It also offers a streamlined process to incorporate other modalities in the future. We introduced two HPT models of different sizes: HPT Pro as our most capable model for solving complex tasks, and HPT Air as our most efficient yet capable model for a wide range of tasks. HPT achieved highly competitive results with the state-of-the-art contenders on multiple benchmarks and has demonstrated impressive multimodal capabilities. Lastly, our HPT Air is publicly available for both research and commercial use.
How to Access HPT
- Open-source release of HPT Air
- Github repo: https://github.com/hyperGAI/HPT
- HuggingFace space: https://huggingface.co/HyperGAI/HPT
- Early Access to HPT Pro prototype/API
- Subscribe to our waitlist to get early access to HPT prototype/API
Explore More
- Contact: Research at hpt@hypergai.com or Business at info@hypergai.com
- Follow us on: LinkedIn, X